일 | 월 | 화 | 수 | 목 | 금 | 토 |
---|---|---|---|---|---|---|
1 | 2 | 3 | 4 | 5 | ||
6 | 7 | 8 | 9 | 10 | 11 | 12 |
13 | 14 | 15 | 16 | 17 | 18 | 19 |
20 | 21 | 22 | 23 | 24 | 25 | 26 |
27 | 28 | 29 | 30 |
- GPT
- GPT-3
- 신뢰성
- AI Fairness
- gpt2
- Ai
- ChatGPT
- 챗GPT
- 딥러닝
- 설명가능성
- nlp
- 챗지피티
- ML
- 케라스
- Bert
- fairness
- 자연어
- 인공지능
- 트랜스포머
- 지피티
- cnn
- trustworthiness
- 인공지능 신뢰성
- MLOps
- 머신러닝
- word2vec
- Tokenization
- DevOps
- Transformer
- XAI
- Today
- Total
research notes
Permutation Feature Importance(PFI) 본문
1. PFI 개요
PFI는 각 feature의 값을 셔플한(shuffled) 후 예측 오류의(prediction error) 증가를 측정한다. PFI 이론은 만약 feature가 target varable와 강한 관계(strong realationship)가 있다면, shuffling 수행에 대한 결과로 예측 오류가 증가 할 것이라는 논리에 기초하고 있다.
만약 feature가 target variable과 강한 관계가 없는 경우에는 예측 오차가 많이 증가하지 않을 것이다. 따라서, 셔플링으로 인해 오류가 가장 많이 증가하는 feature를 기준으로 순위를 매기면 모델의 결과에 가장 많이 영향을 끼치는 feature가 무엇인지 알 수 있다.
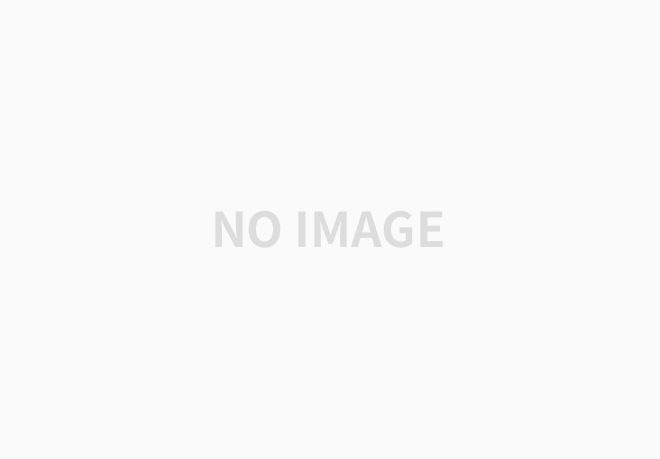
2. PFI 계산 방법
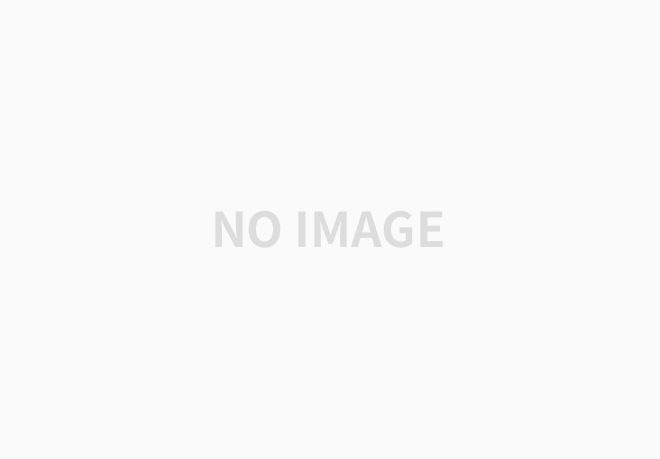
3. Disadvantages
① 통상 레이블이 있는 supervised-learning 에서만 사용가능 (Loss 구할때 필요)
② Permute시 비현실적인 데이터가 발생할 수 있음
③ Permute는 무작위로 섞는 것이기 때문에, 아주 많이 시험하지 않는 이상 feature importance 간 순서가 바뀔 여지가 다분
④ Multicollinearity에 대해 반영하는 방법은 아님
References:
[1] DMQA seminal, 고려대, http://dmqm.korea.ac.kr/activity/seminar/297
[2] https://scikit-learn.org/stable/modules/permutation_importance.html
[3] Serg Masis, Packt publishing, Interpretable Machine Learning with Python
'인공지능 신뢰성 > eXplainable AI(XAI)' 카테고리의 다른 글
DARPA 설명가능성 프로그램 회고 (DARPA’s Explainable AI (XAI) program: A retrospective) (0) | 2022.07.02 |
---|---|
Partial Dependency Plot (PDP plot) (0) | 2022.06.23 |
SHAP (SHapley Additive exPlanations) (0) | 2022.03.16 |
LIME (Linear Interpretable Model-agnostic Explanation) (0) | 2022.02.26 |
Image-specific Saliency (0) | 2022.02.22 |